How and why are we using AI at Sown To Grow?
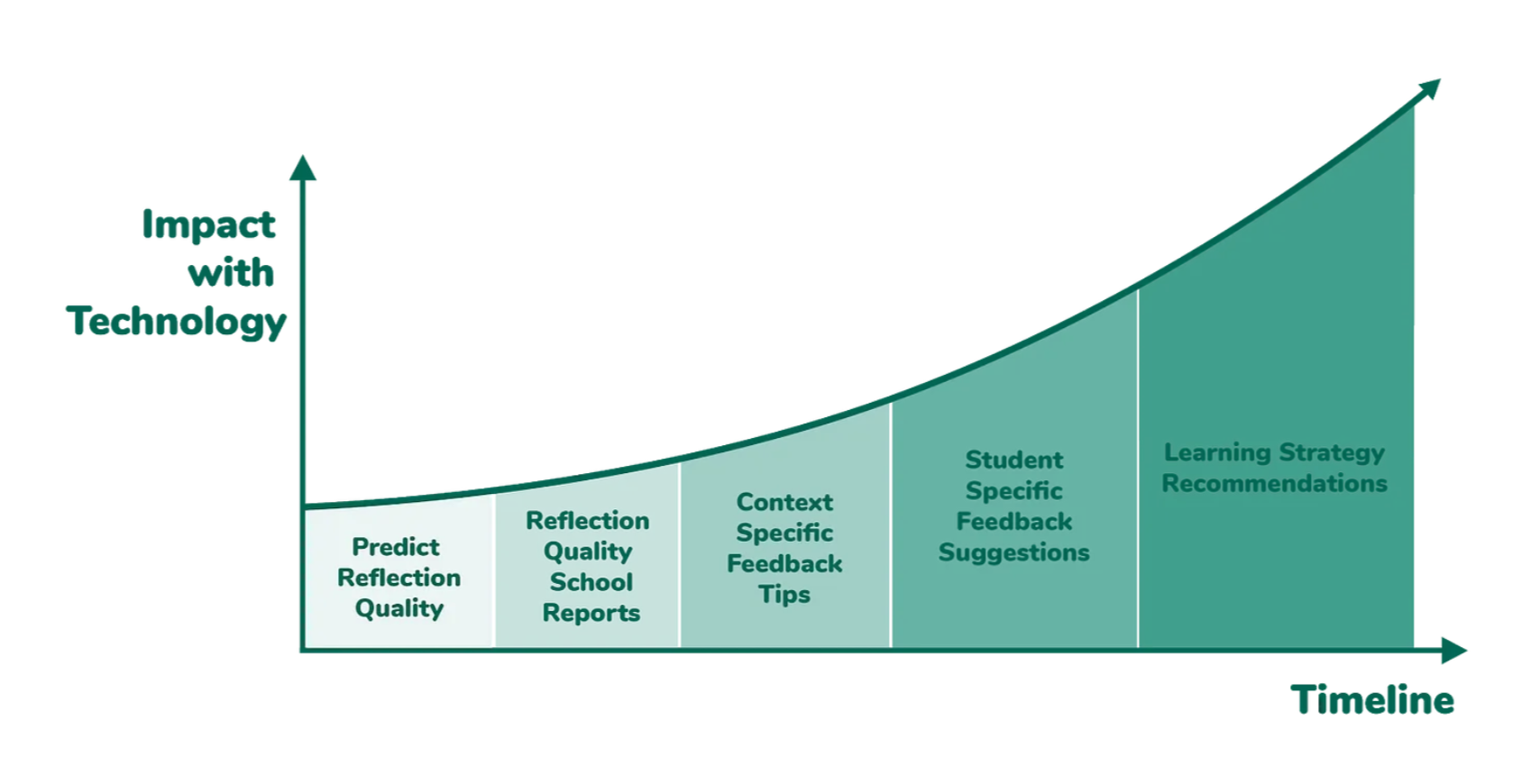
“I barely had time to go to the bathroom during the day” said Francisco, Director of Pedagogy and Partnerships at Sown To Grow, reminiscing his days as a former teacher. I was amazed to see his overwhelmingly busy typical schedule. According to the National Center for Education Statistics, the average U.S. school day is 6.7 hours. With more than half of our team having backgrounds as teachers and school administrators, we are cognizant of the fact that a typical teaching day is 12–16 hours long, and teachers are really busy.
Personalized feedback is important but also time-consuming, hence AI
We know that personalized feedback to students is integral towards learning; however, we also are aware of the unique challenges that teaching brings. While meta-studies from John Hattie tells us feedback is essential towards learning, time constraints is just one of many barriers we recognize. Hence, at Sown To Grow, we aspire to leverage technology, in the form of Natural Language Processing and Machine Learning, to help teachers give meaningful personalized feedback quickly and easily. In this journey (roadmap below), the very first step that we took, with support and validation from National Science Foundation, was to build a machine learning model that scores student’s reflection quality in real time.
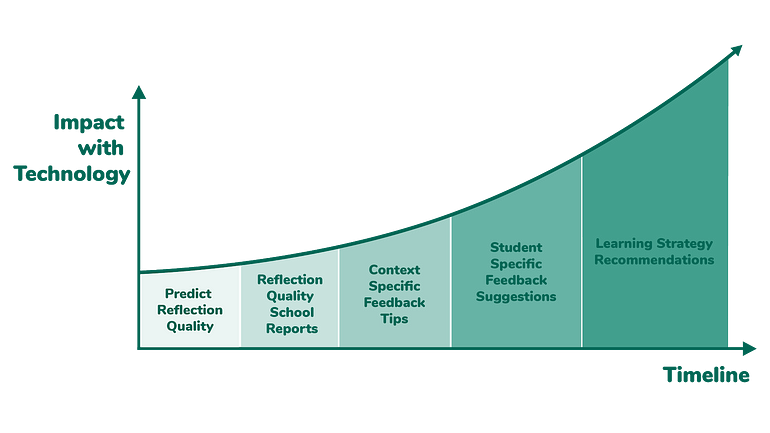
But with great power…
Building any machine learning model is a long and iterative process from accuracy and performance standpoints. Building this model was a particularly deeper process for us because as a team we intended to do it ethically and mindfully. Data, technology and machine learning are powerful. Multiple applications of AI in the forms personalized music and movie recommendations, optimized commute predictions, and spell corrections are already successfully embedded into our day-to-day lives, and the futuristic applications such as self-driving cars are promising.
However, with great power comes great responsibility. There also are as many if not more examples of AI gone bad, or human biases getting reinforced through algorithms if not regulated for. For instance, Amazon’s AI driven recruiting tool was biased against women, and even the tech companies at the forefront of AI are struggling to build algorithms that recognize black faces equally. As quoted by The Brookings Institute, systemic racism and discrimination are already embedded in our educational systems; hence, the implications of AI driven biases in education can be significantly detrimental. As this Forbes article explains, it is important that in classrooms we take a blended approach of teachers plus technology while being vigilant of ethical implications.
Our ethos for applying AI
At Sown To Grow, it is important for us to build artificial intelligence technology with teachers and for teachers. It is in our ethos to make teachers a part of this journey at every point, from helping build our rubrics to scoring reflections for the training data. This series of blogs is another step to bring further transparency to build trust with teachers and have a feedback mechanism from them as we navigate our way to bring ethical AI to the classrooms.
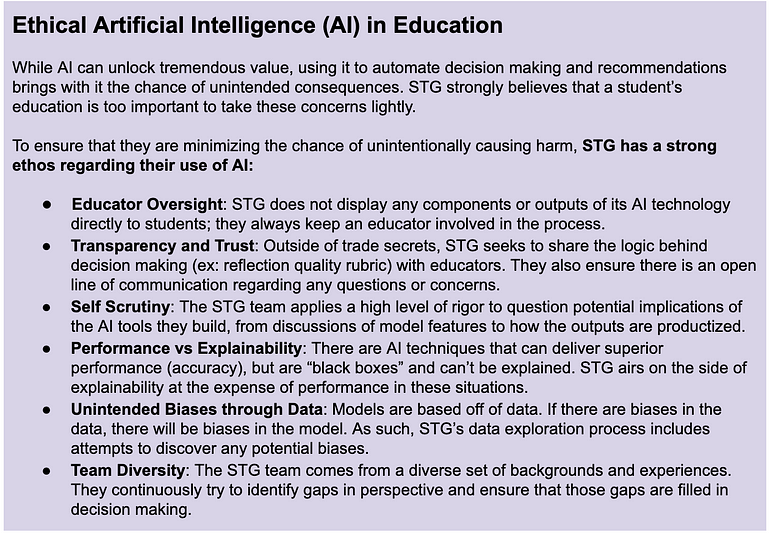
So how does this ML model actually score reflections?
Without getting into the technical nitty-gritty, I would like to introduce you to feature engineering — the secret sauce to create better, accurate and explainable machine learning models.
The very first challenge when we are building a machine learning model from human text is the following:
Students reflect like this:
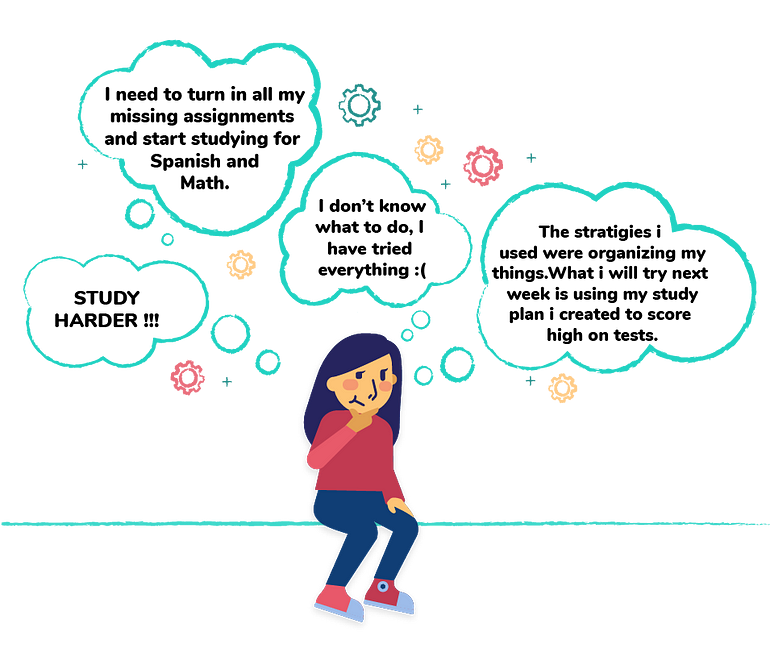
However, machines learn like this:
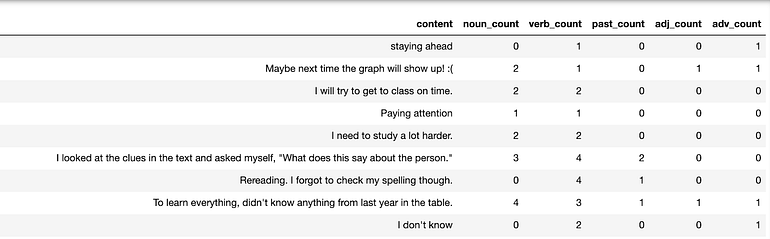
This translation of human language data to machine understandable data is called feature engineering. Features from the text data can be of various types: bag of words (basically just common phrases), word embeddings (quantified relationships between words), count of nouns, verbs, numbers, length of the text, sentiment etc. While features help create better and accurate machine learning models, they also are the primary source for reinforcing unintended bias. For instance, language surrounding a student’s feelings within a reflection when included as a feature in the model improved the accuracy in a statistically significant way. However, that is not one of the features we are using to train the model. Why?
To model a feature or not?
Our goal is for kids to be better reflectors irrespective of how they are feeling, especially when they are not feeling at their best. Even if the data suggests that it is predictive right now, this is a bias we must not reinforce. Similarly, features such as number of capital words, length of the longest word etc. did not make it to the final model despite improving the prediction accuracy because of their potential to reinforce unintended biases based on a student’s language capabilities.
We are working on more ways to make the best out of blended learning, such as allowing teachers to disagree with the model predictions, hence further de-biasing and improving the performance. Stay tuned!
Teachers’ trust in Sown To Grow and its technology is core to us. If you have any feedback, thoughts and ideas, we would love to chat with you.